Machine learning model predicts anastomotic strictures post-surgery
by Hu J, Liu Q (...) Shu Y et 6 al. in Surg Endosc
#Surgery #SurgSky #GeneralSurgery #MedSky
🪡 read our summary
here
📖 read the article:
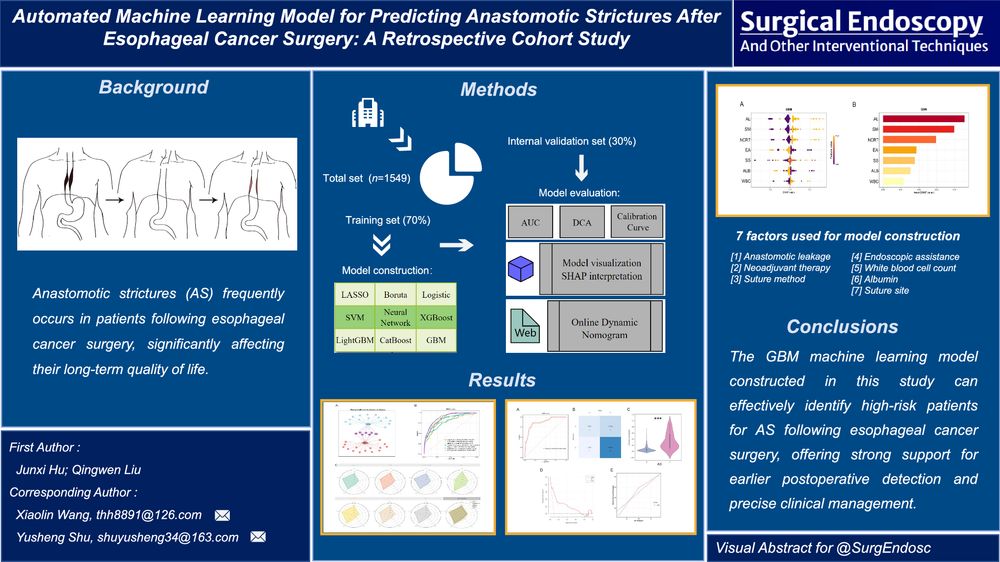
Automated machine learning model for predicting anastomotic strictures after esophageal cancer surgery: a retrospective cohort study - Surgical Endoscopy
Background Anastomotic strictures (AS) frequently occurs in patients following esophageal cancer surgery, significantly affecting their long-term quality of life. This study aims to develop a machine learning model to predict high-risk AS, enabling early intervention and precise management. Methods A total of 1549 patients underwent radical esophageal cancer surgery and were split into a training set (1084) and a validation set (465). Adaptive Synthetic Sampling (ADASYN) handled class imbalance, while Boruta and Least Absolute Shrinkage and Selection Operator (LASSO) with cross-validation refined key features. High-correlation features (r > 0.8) were assessed using variance inflation factors (VIFs) and clinical relevance. Machine learning models were trained and evaluated using area under curve (AUC), accuracy, sensitivity, specificity, calibration curves, and decision curve analysis (DCA). Shapley Additive exPlanations (SHAP) analysis improved model interpretability. Results Seven critical variables were finalized, including anastomotic leakage (AL), neoadjuvant therapy (NCRT), suture method (SM), endoscopic assistance (EA), white blood cell count (WBC), albumin (Alb), and Suture site (SS). The Gradient Boosting Machine (GBM) model achieved the highest AUC, with 0.886 in the training set and 0.872 in the validation set. Shapley Additive Explanations (SHAP) analysis indicated that AL, SM, and NCRT were the most significant variables for model predictions. Conclusion The GBM machine learning model constructed in this study can effectively identify high-risk patients for AS following esophageal cancer surgery, offering strong support for earlier postoperative detection and precise clinical management. Graphical abstract